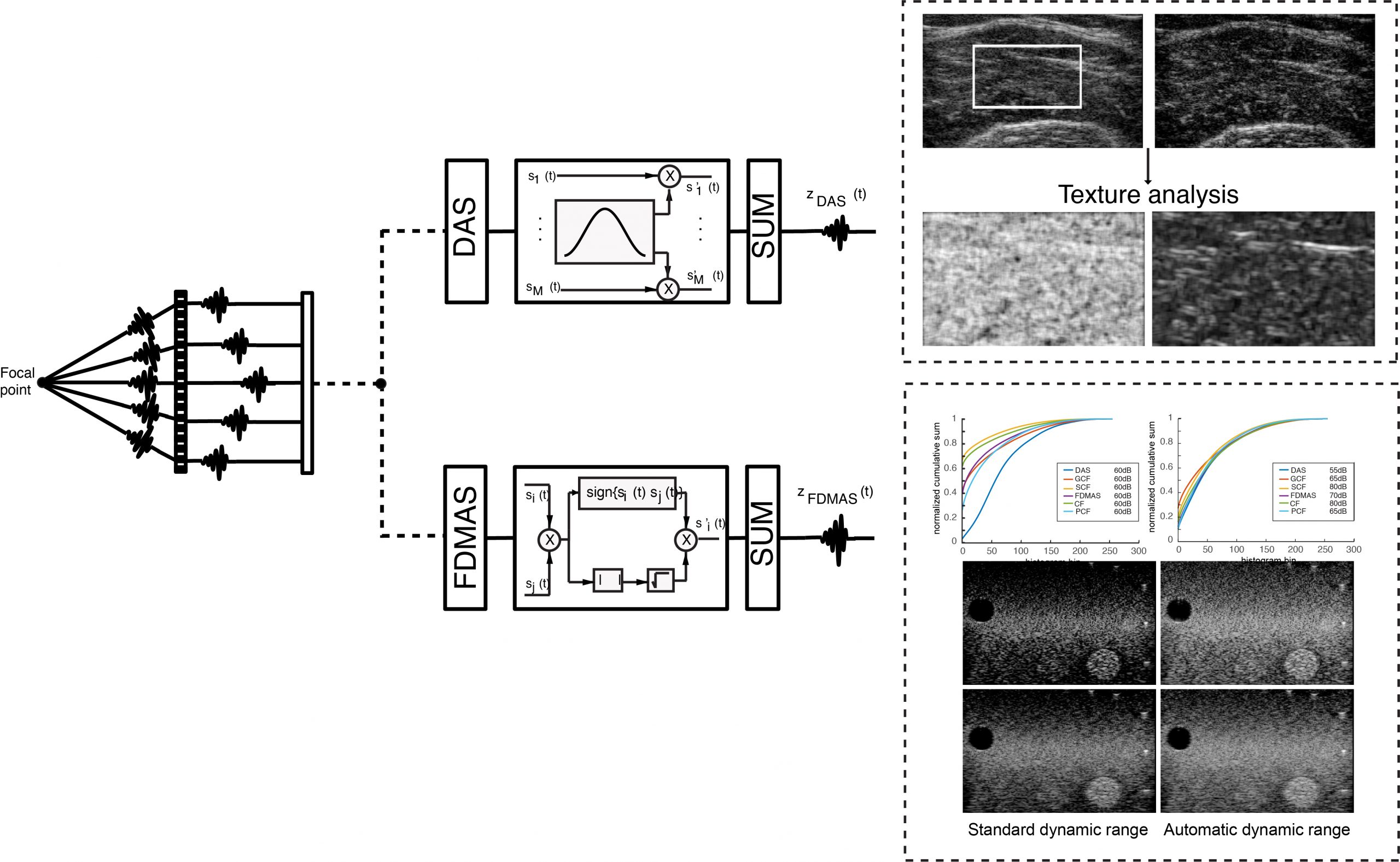
Topic:
Ultrasound imaging can be effective for analysing pathological and normal tissues, by studying both the structure and texture in B-mode images. The output B-mode image is influenced by several technical aspects during acquisition, such as the probe frequency, the dynamic range, and the method used to form the B-mode image, known as beamforming. Numerous beamforming methods exist for ultrasound B-mode imaging, such as filtered delay-multiply-and-sum (FDMAS), but it is known that adaptive/nonlinear beamformers may alter the image quality. Hand-crafted texture features, or automatically learned features by convolutional neural networks (CNNs), are often used on ultrasound images in various applications to give important clinical information. The effects of the beamforming method on the pixel intensity spatial distribution (texture) is currently unknown. Moreover, to obtain the final 8-bit B-mode image that is used for further processing, it is necessary to define a specific dynamic range, which may vary between beamforming methods in order to obtain a visually similar image.
This research line is focused on the robustness of texture features using different beamforming methods, and on the automatic determination of the best dynamic range that optimizes the quality of the B-mode images.