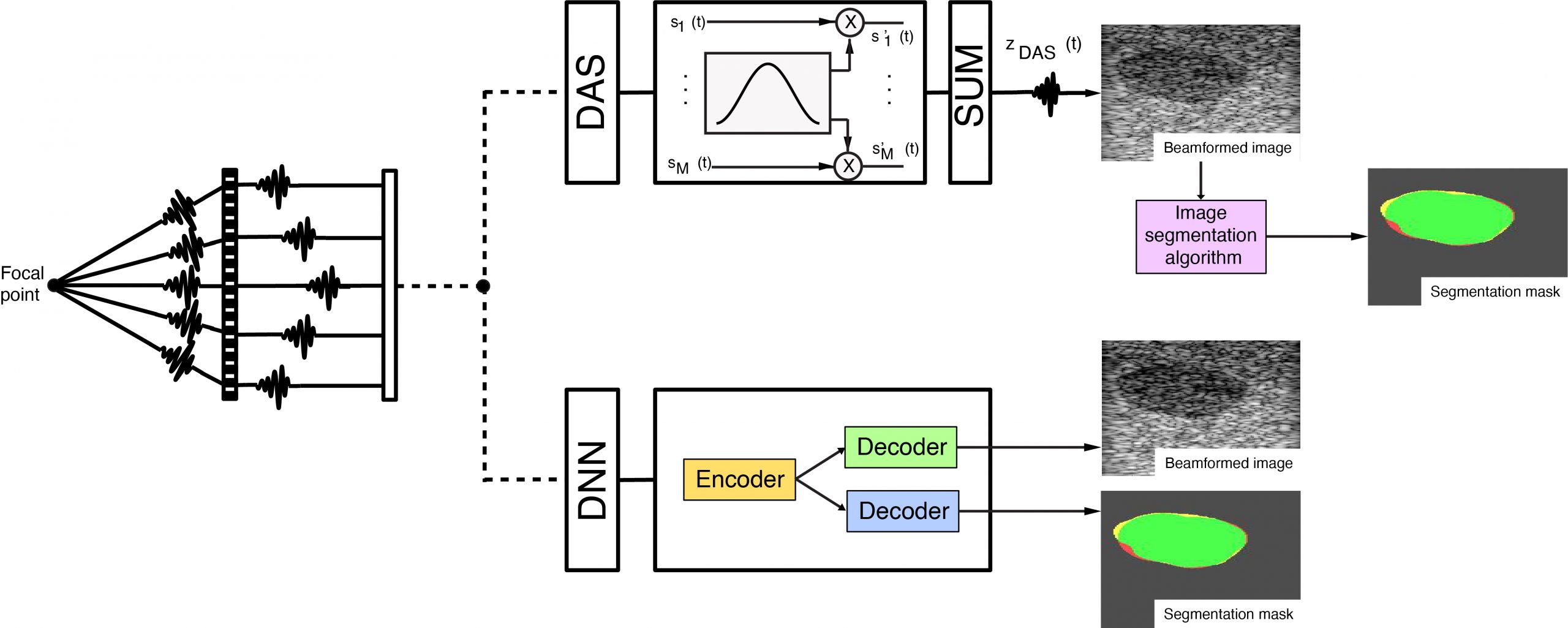
Topic:
Ultrasound imaging can be effective for analysing pathological and normal tissues, by studying both the structure and texture in B-mode images. The output B-mode image is influenced by several technical aspects during acquisition, such as the probe frequency, the dynamic range, and in particular the method used to form the B-mode image, known as beamforming. The beamforming method uses the raw radio frequency signals (RFs) and combines them, influencing the quality of the image, and in particular the resolution and the contrast. The traditional beamforming technique is known as delay and sum (DAS), but several other beamforming algorithms have been proposed and tested, such as filtered delay-multiply-and-sum (FDMAS), and recently, the use of deep learning networks (DNNs) have been proposed. This research line is focused on the extraction of information by the raw channel data, using deep learning networks (DNNs) for both the beamforming process as an alternative to the DAS method, and for simultaneously generating a segmentation mask of structures of interest. The DNNs can successfully translate features learned on Field-II simulated images to phantom and in-vivo data, providing the important benefit of producing multiple outputs from a single input, which is fundamental for parallel clinical and automated decision making.