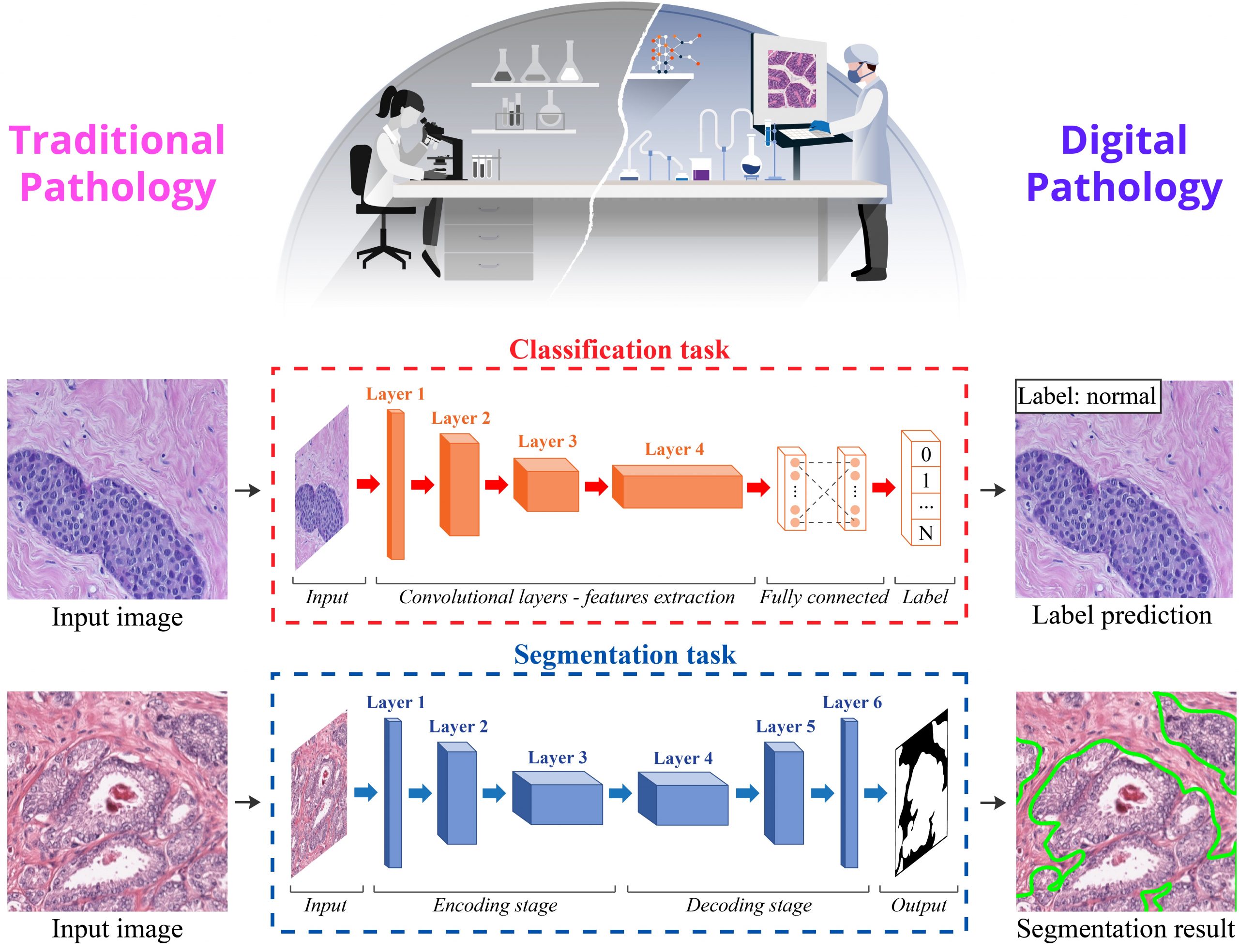
Topic:
The analysis of histological slides is fundamental for cancer diagnosis and grading, typically performed by an expert pathologist, and this process is becoming more and more complex due to the rise in cancer incidence and patient-specific treatment options. Digital pathology (DP) is a sub-field of pathology that focuses on data management based on information generated from digitalized specimen slides. The field of DP is growing and has applications in diagnostic medicine, with the goal of achieving efficient and cheaper diagnoses, and prediction of diseases.
Recently, deep learning (DL) frameworks have rapidly become the main methodology for analyzing medical images. Due to their powerful learning ability and advantages in dealing with complex patterns, DL algorithms are ideal for image analysis challenges, particularly in the field of digital pathology. This research line is focused on the development of automated solutions for digital pathology image analysis. The variety of tasks performed in the context of DP includes classification (e.g., healthy vs. cancerous tissue), detection (e.g., lymphocytes and mitosis counting), segmentation (e.g., nuclei and glands segmentation) and correlation with clinical outcomes (e.g., treatment response).